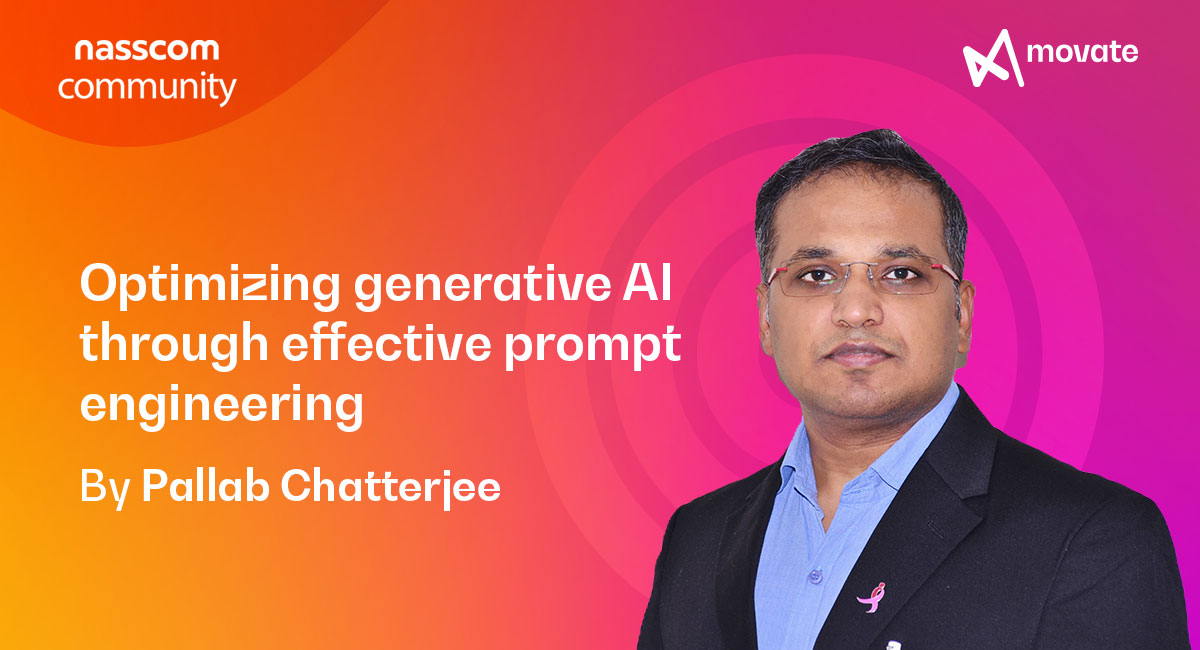
- Media: nasscom
- Spokesperson: Pallab Chatterjee
Generative AI (GenAI), particularly models powered by Large Language Models (LLMs), offers transformative potential across industries. However, without proper planning, the operational costs associated with deploying these models can escalate quickly. This article demonstrates how prompt engineering drives significant cost savings while delivering highly customized outputs for various use cases. This article will also provide a comprehensive analysis of LLM models, their pricing structure, and the operational cost implications. Additionally, it outlined strategies for optimizing expenses, fine-tuning model attributes, and implementing guardrails for efficient operations. This article also addresses the security implications of prompt engineering and introduce specific techniques that enable businesses scale GenAI in a financially sustainable way.
Introduction
GenAI, powered by LLMs such as OpenAI’s GPT series, Google’s PaLM, Anthropic’s Claude, and Meta’s Llama has revolutionized industries by enabling machines to generate text, code, and even creative outputs. These models are potent but resource-intensive, and their improper use can lead to skyrocketing operational costs.
Prompt engineering has emerged as a vital discipline that can help businesses leverage LLMs effectively and efficiently, balancing both performance and cost. This paper will explore how careful manipulation of prompts can reduce costs while maintaining high-quality outputs.
A Quick Overview of LLM Pricing Model
LLMs are neural networks trained on extensive datasets. These models operate by predicting the next token in a sequence based on the input text or “prompt”. Model performance scales with factors such as the number of model parameters, the length of the input context, and the volume of training data. The cost of utilizing LLMs is typically influenced by several factors:
- Number of tokens processed (both in input and output)
- Model size (larger models cost more to use)
- API call frequency
LLM pricing structures differ across service providers and are primarily driven by usage metrics. Below is an analysis of current pricing models and a comparison of associated charges as of the time of writing. It is crucial to acknowledge that these costs are subject to fluctuation, and future readers—especially those reviewing this article six months or more after publication, may observe difference in unit pricing.