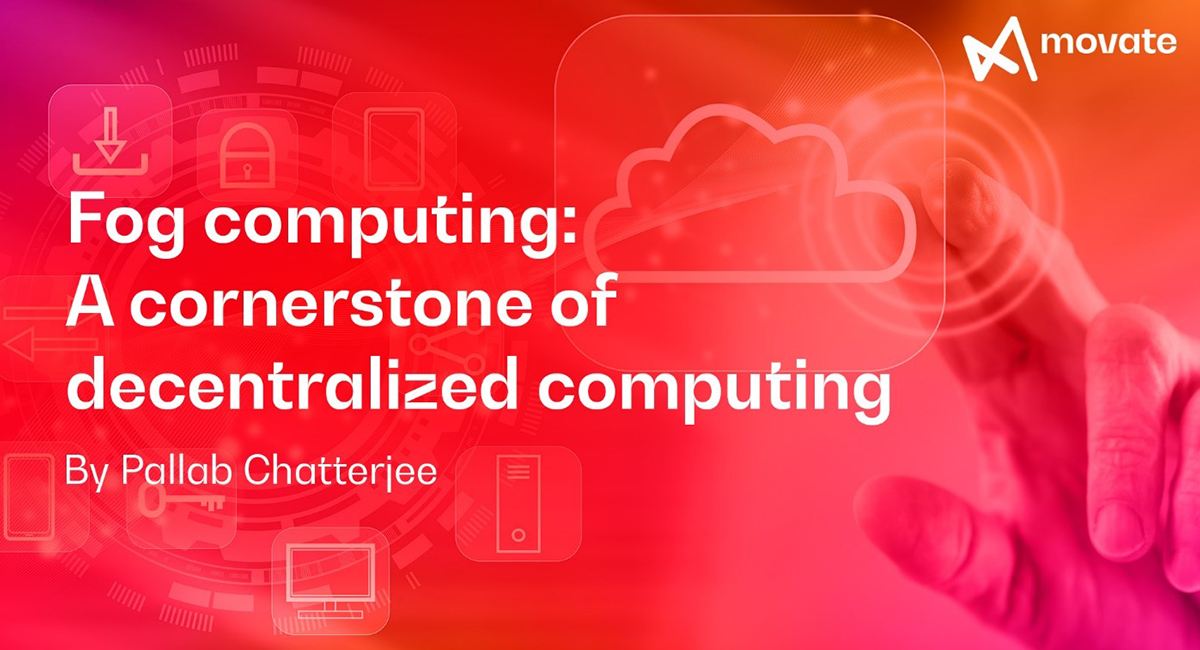
Amidst the surge in data from IoT devices, including sensors and wearables, traditional methods shuttle data to the cloud, causing latency and network congestion. Although edge computing brings computation closer to data sources, it grapples with computational limitations, especially for machine learning tasks. Fog Computing emerges as a transformative solution, extending cloud capabilities to the network edge.
This blog explores Fog Computing’s intricacies, covering key principles, architectural considerations, and potential applications. Unraveling decentralization, it illuminates how Fog Computing revolutionizes data processing, storage, and communication. This paradigm shift promises enhanced efficiency, reduced latency, and unparalleled scalability. Embark on this insightful journey into the future of decentralized computing as we explore the transformative potential of Fog Computing.
Introduction to Fog Computing
Fog Computing, a term coined by Cisco, is a compelling paradigm in the realm of data processing and network architecture. It serves as a connection between edge devices and the cloud, decentralizing data processing by bringing computation closer to the data source. This proximity reduces latency, conserves bandwidth, and enhances the efficiency of data processing, thereby providing real-time insights and faster decision-making capabilities.
Fog computing is especially useful in situations like industrial automation, healthcare monitoring systems, and driverless cars when quick decisions must be made. These systems may react to events in milliseconds by processing data locally, which is not possible with typical cloud computing because of the inherent latency involved in transporting data to and from the cloud.
However, the implementation of Fog Computing is not without its challenges. Issues such as security, scalability, and standardization pose significant hurdles. In the sections below, we dive deeper into the workings of Fog Computing, its applications, and potential solutions to these challenges.
Fog Computing Implementation Details
Fog Computing and Edge Computing, though often used interchangeably, exhibit nuanced differences. While Edge Computing concentrates on nodes in proximity to IoT devices, Fog Computing encompasses resources situated anywhere between the end device and the cloud. Fog Computing introduces a distinct computing layer that employs devices such as M2M gateways and wireless routers, referred to as Fog Computing Nodes (FCN). These nodes play a crucial role in locally computing and storing data from end devices before transmitting it to the Cloud.
- Implementation Architecture:
Fog Computing architecture consists of following three layers:
- Thing Layer: The bottom-most layer, also referred to as the edge layer, constitutes devices such as sensors, mobile phones, smart vehicles, and other IoT devices. Devices in this layer generate diverse data types, spanning environmental factors (e.g., temperature or humidity), mechanical parameters (e.g., pressure or vibration), and digital content (e.g., video feeds or system logs). Connectivity to the network is established through an array of wireless technologies, including Wi-Fi, Bluetooth, Zigbee, or cellular networks. Additionally, some devices may utilize wired connections.
- Fog Layer: The fog node, a crucial and essential part of the fog computing architecture, is at its core. Fog nodes can be virtual or physical, such as virtualized switches, virtual computers, and cloudlets, or they can be gateways, switches, routers, servers, and other physical or virtual components. These nodes are vital in providing the necessary processing power to enable smart end devices to access networks, to which they are tightly connected. The FCNs, whether they are virtual or actual, are diverse. The variety found in FCNs makes it possible to accommodate devices that operate at various protocol layers and makes it easier for the FCN and end device to communicate with non-IP based access technologies.
- Cloud Layer: This is the top-most layer that consists of devices providing large storage and high-performance servers. This layer performs computation analysis and stores data permanently.
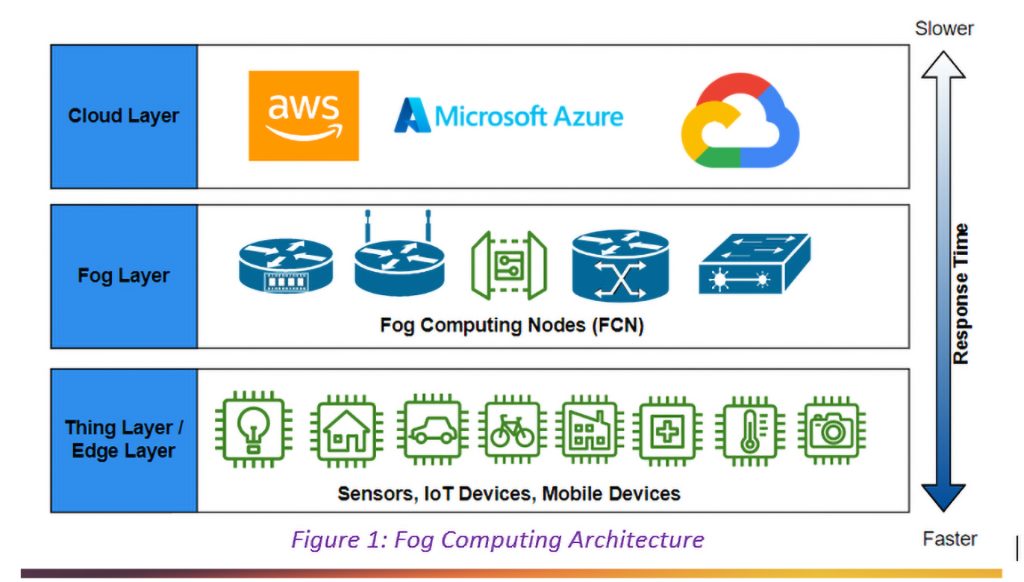
- Request Handling:
The heterogeneous nature of Fog Computing Nodes (FCNs), which accommodates devices operating at different protocol layers and supports a variety of access technologies, is leveraged by Fog Computing’s decentralized infrastructure. In order to ensure that Fog Computing resources are optimally utilized in response to changing demands, the Service Orchestration Layer dynamically assigns resources based on requirements stated by the user.
When end-user requests reach the Fog Orchestrator, accompanied by predefined policy requirements, such as Quality of Service (QoS) and load balancing, the Fog Orchestrator meticulously matches these policies with the services offered by each node. It then furnishes an ordered list of nodes, prioritized based on their suitability against the specified policy. This selection considers factors like availability, ensuring seamless alignment with end user requirements. If the request is time-sensitive and requires low latency, such as adjusting the temperature based on local sensor data or identifying threats in real time from security cameras, the Fog node processes the request locally. However, if the request is resource-intensive and not time-bound, it may be more efficient to send the request to the cloud.
The network’s overall performance is improved, latency is decreased, and resource consumption is optimized with this dynamic approach to request handling. The Fog Computing architecture elevates network operations’ responsiveness and efficiency with its intelligent orchestration and localized processing. A logical diagram of Fog Computing’s request handling is shown in Figure 2.
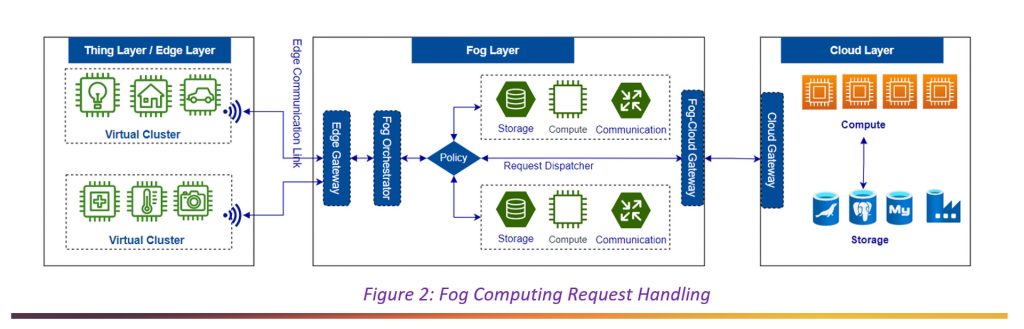
- Data Preprocessing and Contextualization:
Data preprocessing involves collecting, analyzing, and interpreting data at the edge of the network, near the devices that generate the data. Based on the device types and use cases, data may undergo a normalization process, and the processing can continue with or without applying sliding windows. Following data reduction to send the data to the Cloud Layer, two categories of data reduction at the edge are considered – reversible and nonreversible.
- Reversible: By using reduced representations, this method of data reduction allows for the replication of the original data. These methods limit the amount of data that is transmitted over networks and cloud storage by reducing data at the edge. The smaller amount of data can be directly used for machine learning (ML), or the original data source can be replicated first.
- Nonreversible: Nonreversible approaches include those without a way of reproducing the original data after the data have been reduced.
Contextualization in Fog Computing refers to the process of understanding and utilizing the context of data, such as the time, location, and device from which the data originates. By understanding the context, Fog Computing can provide personalized and adaptive services. For example, in a smart home scenario, the fog node can adjust the heating based on the time of day, the presence of people in the house, and the outside temperature.
Illustration of Fog Computing for IoMT applications:
Exploring the intricate operational dynamics of Fog Computing within the realm of Internet of Medical Things (IoMT) applications, let’s delve into the example of a smartwatch, such as the Apple Watch. Packed with sensors like accelerometer, gyroscope, magnetometer, and photoplethysmography, the Apple Watch continuously gathers a wealth of data on various physical activities – steps taken, walking, running, sitting, heart rate, and calories burned. Notably, this data undergoes real-time processing directly on the watch itself, showcasing a prime example of Edge Computing. In scenarios where the heart rate monitor identifies an anomaly, the watch autonomously processes the data locally to instantly alert the user, avoiding the need to transmit it to a remote server.
Now, let’s bring in the concept of Fog Computing, the data storage and processing occur at an intermediary layer, exemplified here by the user’s iPhone, positioned between the cloud data center and other network elements. The watch synchronizes data with the iPhone, enabling more sophisticated processing tasks and detailed analysis of activity data. This information is then transmitted back to the watch. As an illustration, recent watch models allow users to conduct ECG using their Apple Watch, with the processing performed on the connected iPhone to generate graphical representations.
The iPhone can further transmit the data to the cloud (i.e., Apple’s servers) for in-depth analysis, long-term storage, or accessibility on other devices. In summary, utilizing an Apple Watch for activity tracking involves a dual engagement with both Edge and Fog Computing. The watch (Edge) undertakes initial data collection and processing, subsequently collaborating with the iPhone (Fog) for additional processing and synchronization with the cloud.
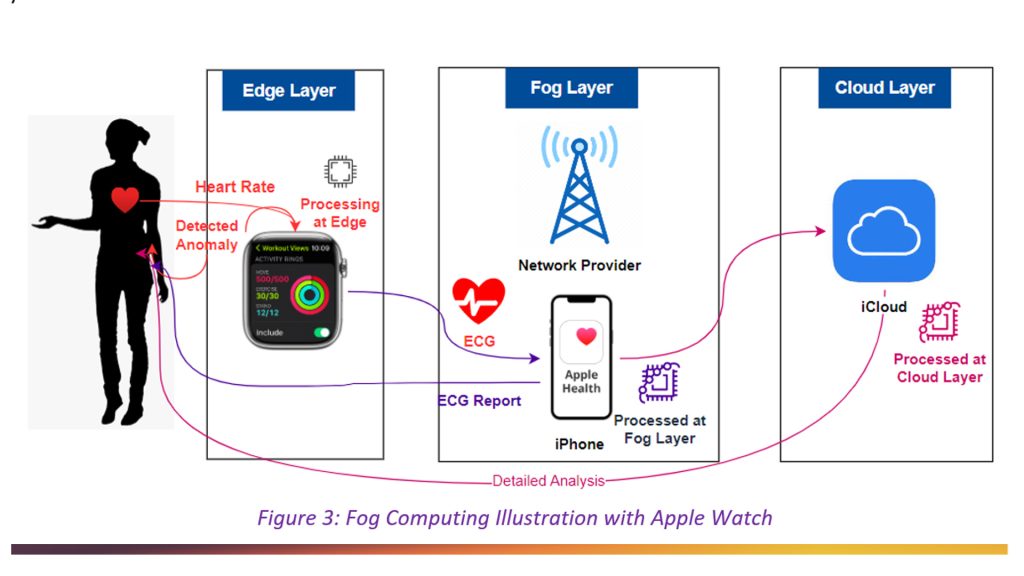
Benefits of Fog Computing:
As a distributed paradigm that is well positioned between cloud computing and the Internet of Things, fog computing is essential. It serves as a smooth link between edge computing, cloud computing, and the Internet of Things. Apart from being a distinguishing characteristic, this well-chosen location offers several advantages that need recognition.
Following are some key benefits:
- Reduced Latency: By processing data in proximity to the source, fog computing can significantly reduce latency, making it the better choice for real-time applications such as autonomous vehicles, telemedicine, and telesurgery.
- Efficient Network Utilization: Fog computing can reduce volumes of data that need to be transmitted to the cloud, alleviating network congestion and improving overall network efficiency.
- Contextual Awareness: The needs and goals of the customer are considered in-depthi during the design of the Fog infrastructure. Along the Cloud-to-Things continuum, this makes it possible to precisely distribute compute, communication, control, and storage capabilities. As a result, apps are created that are incredibly customized to satisfy the unique requirements of customers.
- Operational Resilience: The Fog architecture supports the pooling computing, storage, communication, and control functions across the spectrum between Cloud and IoT. Fog nodes have the capability to function autonomously, independent of the central Cloud layer, providing enhance operational resilience and fault tolerance.
- Improved Privacy and Security: Data can be processed locally within the fog nodes, reducing the need to transmit sensitive information over the network, thereby enhancing privacy and security.
Open Challenges of Fog Computing:
While fog computing offers numerous benefits, it also presents several open challenges that need to be addressed:
- Resource Management: Efficient management of resources in a fog environment is a complex task due to the heterogeneity and geographical distribution of fog nodes. For example, a video streaming application might require high bandwidth and processing power, while a temperature monitoring application might only need minimal resources.
- Standardization: Currently, there are no universally accepted standards for fog computing. The lack of standardization can lead to compatibility issues between different fog systems and services. For example, an IoT device manufactured by one company might not work seamlessly with the fog infrastructure provided by another company.
- Security and Privacy: Fog computing introduces new security challenges. For instance, data stored on a fog node could be physically tampered with if the node is not adequately secured. Additionally, data transmitted between fog nodes could be intercepted if the communication channels are not properly encrypted. A real-life example could be a smart home system, where sensitive data like home security footage needs to be protected.
- Quality of Service (QoS): Ensuring a consistent QoS across a distributed, heterogeneous fog environment is challenging. For instance, an autonomous vehicle relying on a fog computing infrastructure for real-time decision making requires a high level of reliability and low latency. Any inconsistency in service can have serious consequences.
- Energy Efficiency: Fog nodes, particularly those deployed at the edge of the network, often have limited power resources. Therefore, energy-efficient operation is a critical challenge for fog computing. For instance, a fog node deployed in a remote wildlife monitoring station needs to manage its resources efficiently to prolong battery life
Conclusion
Fog computing, a cornerstone of decentralized computing, is poised to reshape our digital landscape. By bringing computation and storage closer to data sources, it transforms how we handle IoT-generated data. Exploring the future through fog computing reveals benefits like reduced latency, enhanced privacy, and efficient network utilization.
Yet, challenges abound. Resource management, security, standardization, quality of service, scalability, and energy efficiency pose hurdles. Addressing these challenges demands ongoing research and innovation. As we delve deeper into decentralized computing, fog computing’s role grows pivotal. It’s a journey of discovery, innovation, and problem-solving. Successfully navigating challenges is key to unlocking fog computing’s potential. This journey promises a more efficient, responsive, and decentralized digital world.
Author’s background and experience
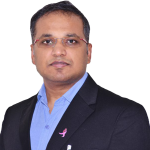
Cloud practices and initiatives are led by Pallab, a Senior Director and Enterprise Solution Architect at Movate. Having worked in many different industries and countries for more than 16 years, he is an expert Multi-Cloud Specialist. Pallab is an expert at orchestrating successful migrations of over 25 workloads across major cloud hyperscalers. He is an expert in edge computing, big data, security, and the Internet of Things. Notably, he has designed more than ten innovative use cases in edge computing, IoT, AI/ML, and data analytics, establishing his standing as a forerunner in the tech industry.
More blogs by Pallab
- Movate’s Virtual Try-On solution for a top brand
- Revolutionizing IT: The Impact of GenAI on Traditional IT Operations and Infrastructure
- Beauty in the Clouds: One of the Global Cosmetics Giant’s Remarkable Metamorphosis with Movate & AWS
- Breaking Barriers: The Voyage of a F50 Organization Towards 25% Operational Savings with Movate and Microsoft Azure
- From Legacy to Leading Edge: A Texan Primary Care Provider’s Journey to 42% OpEx Reduction with Movate and Azure Serverless.
- Debunking Migration Myths: Unveiling the Truths of Hyperscaler Migration with Movate