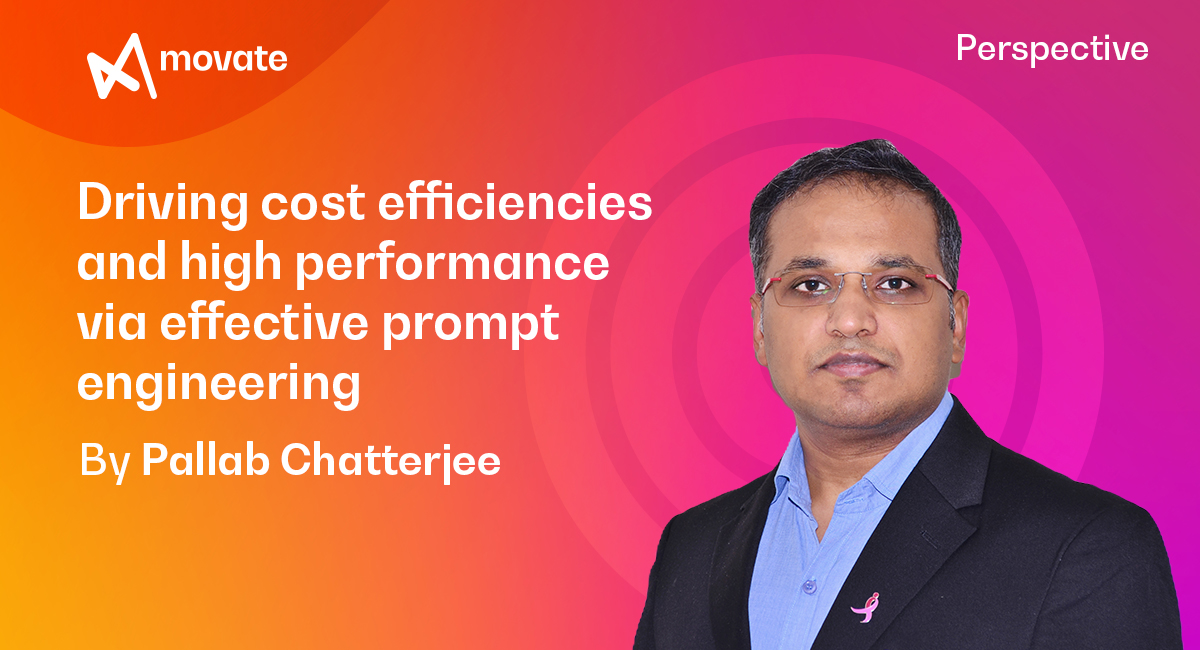
GenAI powered by LLMs offer significant transformational potential across industry use cases. On the flip side, the operational costs associated with deploying these models surges due to improper planning.
This blog delves into the following topics:
- Analysis of LLMs—pricing structures
- Effective prompt engineering
- Basic prompt and an effective prompt
- Factors that escalate operational costs
- Guardrails for efficient operations
- Performance tuning for efficiency
Prompt engineering has emerged as a vital discipline that can help enterprises leverage LLMs effectively and efficiently, balancing both performance and cost. LLMs (Llama, GPT, PaLM, Claude and the rest) have revolutionized enterprises across the board by equipping machines to generate a wide variety of outputs and creative content, but these models are resource intensive. Their improper planning and use can lead to skyrocketing operational costs for businesses
LLM pricing structures
LLMs are neural networks trained on extensive datasets and operate by predicting the next token in a sequence based on the input text or “prompt.” A model’s performance scales with factors such as the number of model parameters, the length of the input context, and the volume of training data. The cost of utilizing LLMs is usually influenced by different criteria such as the API call frequency, the model size (larger the model, higher the cost), and number of input and output tokens being processed.
Pricing structures vary across service providers and are primarily driven by usage metrics.
Note that the models and their respective cost structures outlined in Exhibit 1 are prone to change. The chart is based on information available at the time of publishing this blog.
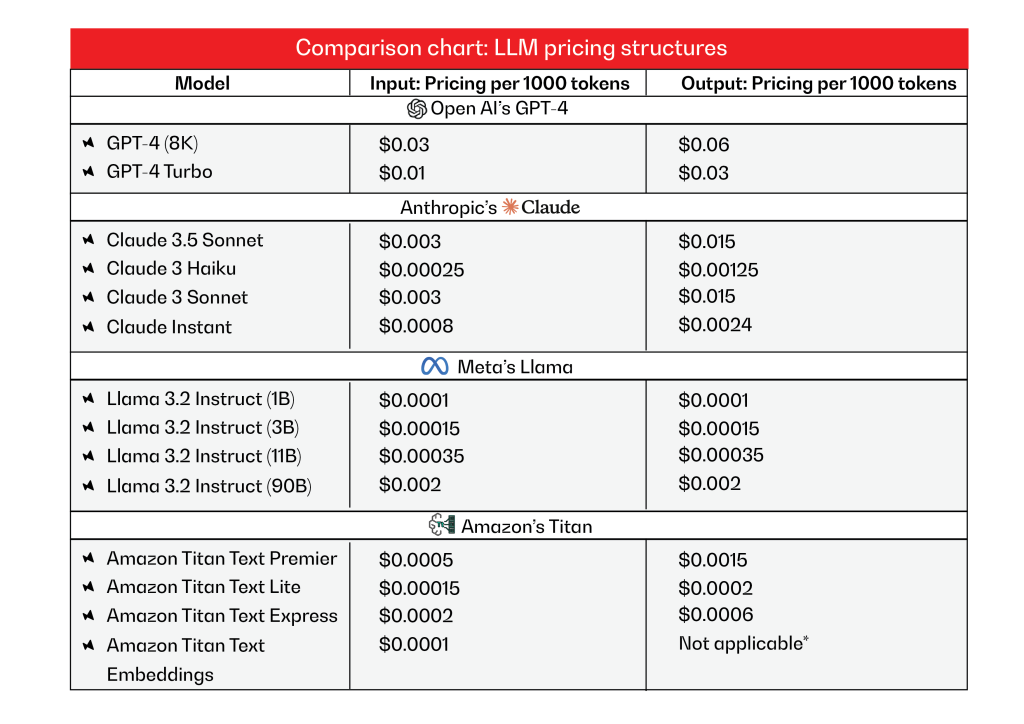
*Not applicable (Exhibit 1) as embeddings don’t generate output tokens like text-based models.
As outlined in Exhibit 1, these examples represent only a subset of available LLM model pricing structures. While the cost per 1,000 input or output tokens may seem negligible, the compounding effect is likely to incur high monthly expenses, particularly for production workloads.
To comprehend the pricing model more thoroughly, it is essential to understand the concepts of input and output tokens. Tokens are fragments of text fed into or generated by the LLM. They can consist of words, characters, or subwords. In this context, input tokens are the text sent to the model, while output tokens are the text produced by the model.
For instance, in the sentence “The quick brown fox,” the input tokens are:
- “The,” “quick,” “brown,” and “fox.”
If the model completes the sentence with “jumps over the lazy dog,” the output tokens would be:
- “jumps,” “over,” “the,” “lazy,” and “dog.”
LLMs typically charge based on the number of tokens processed, involving both input and output. Every token adds up to the total cost.
Effective prompt engineering
Crafting the optimal input text (prompt) invokes the best possible response from an LLM, thus improving output quality and reducing the need for multiple attempts, which can surge costs. Careful prompt engineering for enterprise cost savings matters as it optimizes tokens, improves output quality, accuracy and speed of response, lowers computational load.
- Speed and efficiency: Better prompts can generate more accurate responses faster, leading to faster task completion.
- Improved output quality: Well-crafted prompts reduce the need for re-queries and multiple interactions.
- Token optimization: Every interaction with an LLM is token-based. Efficient prompts reduce token usage, limiting unnecessary charges.
- Reduced resource usage: Optimizing context length and request frequency lowers computational load.
Basic versus effective prompt
Consider this example, where a user needs a quick summary on ‘What is cloud computing?” for a high-school seminar.
A basic prompt a person would usually input would be “What is cloud computing?”
But an effective prompt would be this:
- “Instruction: write a response to explain what cloud is computing.
- Context: I will attend a high-school event to explain cloud computing concepts to 10th graders
- Output Indicator: explain the cloud computing concepts with real-life examples
- Answer shouldn’t exceed more than 100 words.”
Here is the comparison of the output of two different prompts (generic one and the effective one):
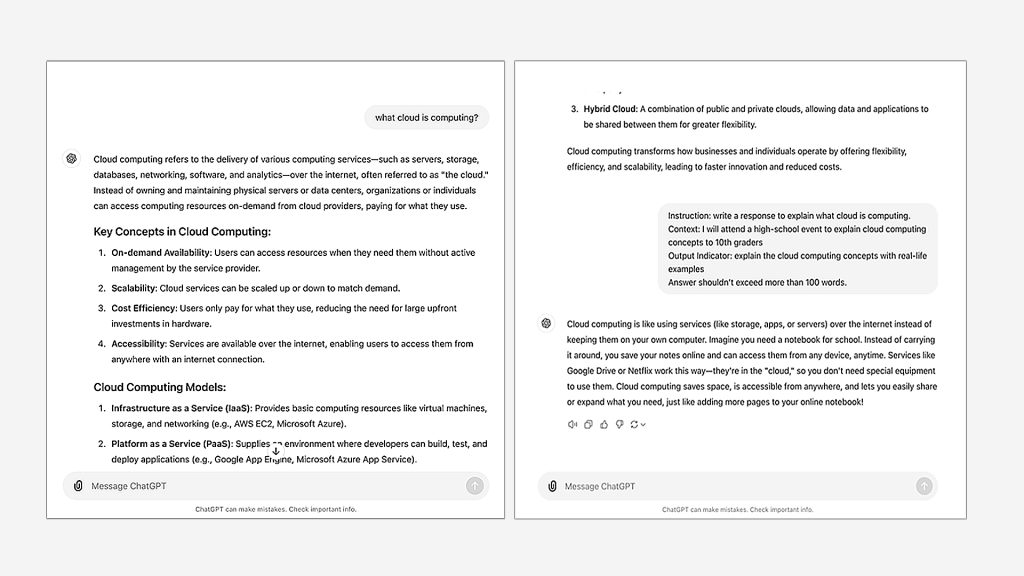
So, what’s in an effective prompt? Specificity, structure, output indicator and negative prompt:
- Specificity: The prompt specifies the target audience (high-school event and 10th graders). This context helps the AI tailor its responses appropriately.
- Structure: The prompt asks for a specific format (explanation with real-life example, answer is not more than 100 words), guiding the AI on how to organize the information.
- Output indicator: The prompt specifies the format and desired tone, helping the AI understand the type of language to use.
- Negative prompt: With the answer not exceeding 100 words, the prompt explicitly instructs the AI model on what not to include or do in its response
Technical specifications include token usage and model efficiency. By limiting the number of tokens in the output response, organization can save costs associated with token processing. A well-crafted prompt ensures the model can quickly understand and generate the desired output, improving overall efficiency.
LLMs: Cost-escalating factors
Let’s look at the model attributes that can drastically surge operational costs unless they’re controlled.
- Token count: Many businesses overlook token limits. Each API request typically has a token cap (e.g., GPT-4 caps at 8k to 32k tokens per query). Exceeding token limits forces truncation or necessitates multiple requests, escalating costs.
- Model size: Using larger models (e.g., GPT-4 over GPT-3) is more expensive, even for simple queries. Businesses must select the right model based on their requirements.
- Request frequency: Repeated queries, especially poorly designed ones, can cause an accumulation of token charges. It’s crucial to ensure that each request maximizes the value extracted from the model.
- Context management: In conversation-based models, maintaining too long of a context window increases the number of tokens used in each request. It’s advisable to trim unnecessary context.
- Iteration loops: Retraining or fine-tuning LLMs without refining the scope or goals can lead to unnecessary compute and storage costs.
Guardrails for efficient operations
Key guardrails to rein in costs include the token limits, model selection, refining queries and context pruning.
- Token limits: Monitor token usage per request. Set hard limits on the number of tokens per API call to avoid unnecessary processing.
- Model selection: Opt for smaller models when applicable. Use larger models only when the complexity of the task justifies it.
- Frequency management: Reduce the number of queries by refining each one with effective prompt engineering.
- Context pruning: Regularly trim the conversation or context history to prevent unneeded token consumption.
These guardrails help enterprises to scale LLM usage in a financially viable manner that meets compliance standards and optimizes performance.
Performance tuning for efficient operations
To balance cost-efficiency with high operational performance, implement the following strategies:
- Model tuning: Experiment with lower temperatures (for more predictable outputs) or adjust top-p and top-k parameters to control randomness in responses.
- Throttling: Set API throttling limits to avoid exceeding budgetary constraints.
- Resource autoscaling: Implement autoscaling solutions to ensure computing resources are dynamically allocated based on workload demand.
- Custom training: Instead of using the largest available model, custom-train smaller models on domain-specific data to improve relevance while reducing costs.
Proper cost-control measures, performance tuning, and security protocols ensure that enterprises get the maximum value out of their GenAI deployments.
No doubt GenAI presents opportunities and benefits. While on one hand benefiting from LLMs’ versatility and prowess, on the other hand, enterprise operational leaders needn’t open a pandora’s box. Through effective prompt engineering, they can boost output quality, reduce token usage, and optimize costs.
Author’s background and expertise
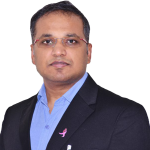
Pallab leads the cloud practice at Movate. Having worked in many different industries and countries for more than 16 years, he is an expert Multi-Cloud Specialist. Pallab is an expert at orchestrating successful migrations of over 25 workloads across major cloud hyperscalers. He is an expert in edge computing, big data, security, and the Internet of Things. He has designed more than ten innovative use cases in edge computing, IoT, AI/ML, and data analytics, establishing his standing as a forerunner in the tech industry. LinkedIn